The recent surge of excitement surrounding generative artificial intelligence (AI) has set high expectations for supply chain leaders to harness AI and other advanced technologies, driving increased efficiency and growth within their organizations.
However, this wave of excitement has also highlighted the fact that many supply chain planning processes are yet to establish a strong foundation for leveraging advanced AI solutions. This includes both emerging AI solutions and established planning tools that already integrate AI capabilities. The good news is that supply chain planning is well-suited for experimenting with various AI and machine learning (ML) use cases. Taking small steps in the right direction can accumulate into significant results, but the key is to begin now.
Even before the recent buzz around technologies like ChatGPT and generative AI, supply chain leaders consistently ranked data-centric AI and ML as their top digital priority among the technologies they are testing or deploying. This insight comes from Gartner’s Digital Business Impact on Supply Chain Survey conducted between March and April 2022.
The intention to implement AI and ML is evident. However, supply chain leaders face a dual challenge when it comes to implementation. They need to enhance their existing data management practices to effectively harness AI and ML technologies, all while identifying the right opportunities to integrate these solutions into their planning processes.
For leaders grappling with these challenges, now is the time to take action. Supply chain organizations that don’t adopt AI and ML-supported planning processes will fall behind in competitiveness, struggling to gain insights into market dynamics for agile decision-making.
Finding the Right Entry Point for AI and ML
When introducing AI and ML technology, avoid overcomplicating your initial implementation. Instead, explore leveraging existing planning analytics technology that doesn’t require extensive system development or data work. This approach minimizes financial risks, delivers initial automation benefits, and builds momentum within the organization for further investment and deployment. Systems already established within your organization also provide an excellent learning environment for training planners.
A solid entry point for AI and ML implementation is to support ongoing data quality validation and management. This includes tasks such as cleansing sales and operational history data, analyzing and aligning product relationships. Beyond data validation and cleansing, existing solutions may present clear opportunities for planners to become more comfortable with decision automation. To identify potential use cases for greater decision automation, focus on what data is most valid and helpful and verify if it is readily available.
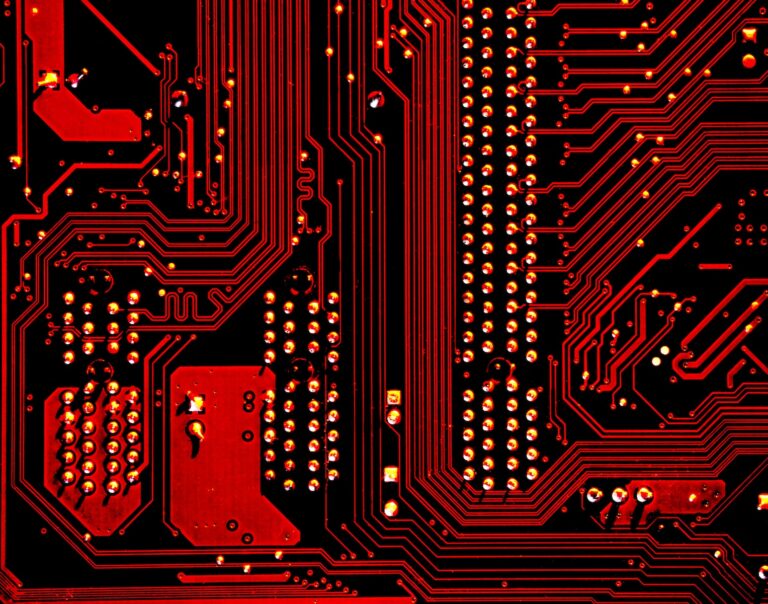